A multi-index learning approach for classification of high-resolution remotely sensed images over urban areas |
| |
Affiliation: | 1. Institute of Agricultural Resources and Regional Planning, Chinese Academy of Agricultural Sciences/Key Laboratory of Agricultural Remote Sensing, Ministry of Agriculture, P. R. China, Beijing 100081, China;2. Exploration and Production Research Institute, SINOPEC, Beijing 100083, China;3. Institute of Environment and Sustainable Development in Agriculture, Chinese Academy of Agricultural Sciences, Beijing 100081, China;4. State Key Laboratory of Resources and Environmental Information System/Institute of Geographic Science and Natural Resources Research, Chinese Academy of Sciences, Beijing 100101, China |
| |
Abstract: | 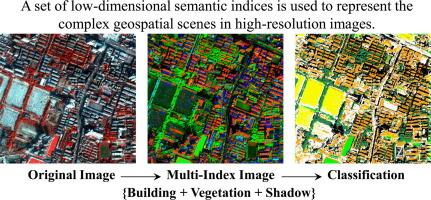 In recent years, it has been widely agreed that spatial features derived from textural, structural, and object-based methods are important information sources to complement spectral properties for accurate urban classification of high-resolution imagery. However, the spatial features always refer to a series of parameters, such as scales, directions, and statistical measures, leading to high-dimensional feature space. The high-dimensional space is almost impractical to deal with considering the huge storage and computational cost while processing high-resolution images. To this aim, we propose a novel multi-index learning (MIL) method, where a set of low-dimensional information indices is used to represent the complex geospatial scenes in high-resolution images. Specifically, two categories of indices are proposed in the study: (1) Primitive indices (PI): High-resolution urban scenes are represented using a group of primitives (e.g., building/shadow/vegetation) that are calculated automatically and rapidly; (2) Variation indices (VI): A couple of spectral and spatial variation indices are proposed based on the 3D wavelet transformation in order to describe the local variation in the joint spectral-spatial domains. In this way, urban landscapes can be decomposed into a set of low-dimensional and semantic indices replacing the high-dimensional but low-level features (e.g., textures). The information indices are then learned via the multi-kernel support vector machines. The proposed MIL method is evaluated using various high-resolution images including GeoEye-1, QuickBird, WorldView-2, and ZY-3, as well as an elaborate comparison to the state-of-the-art image classification algorithms such as object-based analysis, and spectral-spatial approaches based on textural and morphological features. It is revealed that the MIL method is able to achieve promising results with a low-dimensional feature space, and, provide a practical strategy for processing large-scale high-resolution images. |
| |
Keywords: | High spatial resolution Classification SVM Morphological Texture Feature extraction |
本文献已被 ScienceDirect 等数据库收录! |
|